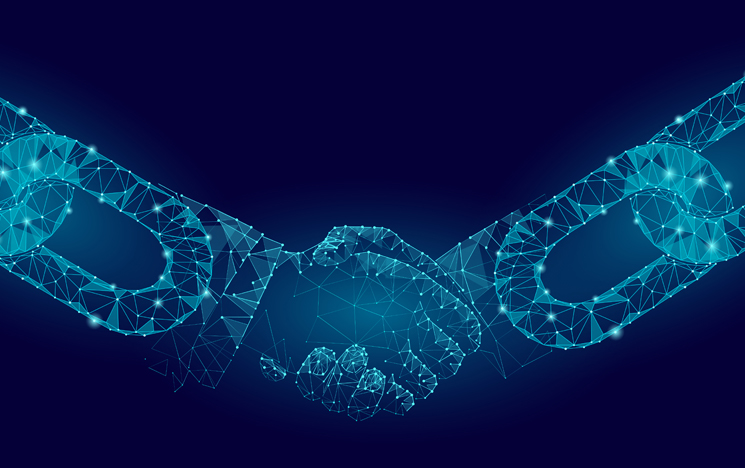
Quantitative FinTech (QFIN)
The Quantitative FinTech (QFIN) research group at Sussex provides independent, customized and responsible solutions to promote innovation in financial markets. Our flexible approach resolves problems ranging from small boutique model development to advice on implementing complex financial systems.
QFIN aims to provide excellent research on issues currently faced by financial markets and promote stronger links between academic institutions, business and industries.
QFIN is one of two research groups in the Department of Accounting and Finance in the University of Sussex Business School. We aim to provide excellent research on issues currently faced by financial markets (including digital assets and their derivatives such as bitcoin swaps, futures and options, climate change finance and risk management). The problems that our researchers study require a data-driven quantitative approach including the analysis of big data sets derived from trade or order book data at ultra-high frequency.
QFIN has experts in quantitative finance, climate change, crypto asset market microstructure, big data analysis, machine learning and computer science. The network’s core and key associated members all have strong research backgrounds with publications in the top academic journals and some also have significant industry experience having held various roles in top-tier investment banks, hedge funds and relevant industries.
QFIN aims to promote stronger links between academic institutions and business and industries. We work and collaborate with business and industry on research initiatives and projects. We provide bespoke consultancy services, design and deliver tailor-made training courses as well as teach students to meet the challenges for a highly-skilled labour force that the industry requires.
Research Seminars
Wednesday 1 February: Sam Rosen – Temple University
Investor Experience Matters: Evidence from Generative Art Collections on the Blockchain
Wednesday 8 February: Marc-Oliver Pohle – Goethe University, Frankfurt
Generalised Correlation
Wednesday 15 February: Grigory Vilkov – Frankfurt School of Finance and Management
Media Narratives and Price Informativeness
Wednesday 22 February: Jie Cao – Hong Kong Polytechnic University
Forecasting Option Returns with News
Wednesday 8 March: Bing Han - Rotman School of Management, University of Toronto
Idiosyncratic Volatility and the ICAPM
Wednesday 22 March: Carole Bernard – Grenoble Ecole de Management
Option-Implied Dependence and Correlation Risk Premium
Wednesday 29 March: Raman Uppal – EDHEC Business School
What is Missing in Asset-Pricing Factor Models
Visit the Business School's Research Events page to register for any of the above Spring 2023 QFIN seminars.
Highlights of Recent Research
Alexander, C., Deng, J., and Zou, B. (2022) Hedging with automatic liquidation and leverage selection on bitcoin futures. European Journal of Operational Research. pp. 1-16. ISSN 0377-2217.
Alexander, C., Deng, J., Feng, J. and Wan, H. (2022) Net buying pressure and the information in bitcoin option trades. Journal of Financial Markets. a100764. ISSN 1386-4181.
Alexander, C., Han, Y., and Meng, X. (2022) Static and dynamic models for multivariate distribution forecasts: proper scoring rule tests of factor-quantile vs. multivariate GARCH models. International Journal of Forecasting. pp. 2-19. ISSN 0169-2070.
Alexander, C., Meng, X., and Wei, W. Targetting Kollo skewness with random orthogonal matrix simulation. European Journal of Operational Research, 299 (2022): 362-376.
Alexander, C. and M. Dakos (2022). Assessing the accuracy of exponentially weighted moving average models for Value-at-Risk and Expected Shortfall of crypto portfolios. Quantitative Finance. ISSN 1469-7688.
Barunik, J., Bevilacqua, M., and Tunaru, R. (2022). Asymmetric network connectedness of fears. Review of Economics and Statistics, 104(6), 1304-1316.
Kaeck, A., van Kervel, V., and Seeger, N. J. (2022). Price impact versus bid–ask spreads in the
index option market. Journal of Financial Markets, 59, 100675.
Meng, X. and Taylor, J. W. Comparing probabilistic forecasts of the daily minimum and maximum temperature. International Journal of Forecasting, 38 (2022): 267-281.
Contact us
Email: qfin@sussex.ac.uk