Dr Dhruva Raman
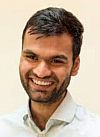
Computational modelling of neural circuits
We're broadly interested in 'systems-level' biological questions. How does a (neural circuit/metabolic pathway/...) organise and adapt to achieve precise, varied functional goals, while coupled to the rest of the body as well as a dynamic environment?
What's our approach? For an explanation by example, I'll summarise one current project focusing on the insect mushroom body, a brain structure that organises associative learning in insects. I’ll use this to introduce more generally the type of project that interests me. You could be involved in the mentioned project, or another, depending on your preferences.
The mushroom body must navigate an unavoidable tradeoff. On the one hand, learning can be improved by usefully incorporating past data. On the other hand, over-reliance on past data makes learning vulnerable to data scarcity and/or environmental shifts that reduce the salience of past experience. Since insects have short lifespans and simplified models of the environment, both of these vulnerabilities are relevant. We've built design principles for a system that can flexibly weight either side of this tradeoff, and are using these to understand and predict the organisation and dynamics of the mushroom body across learning scenarios. We will then ask how these insights translate to, and differ in, less experimentally accessible mammalian brain structures (the cerebellum).
More generally, we use mathematics and computation to express how competing functional goals must constrain system design. We simultaneously observe the design of biological systems (in collaboration with experimenters) to better understand their functional goals. Finally, we look for design principles that lead to experimentally testable hypotheses. We favour comparative hypotheses that explain variation across animals and species in terms of differing functional goals. We favour hypotheses that depend on principles and tradeoffs, rather than highly specific algorithmic implementations of learning and function.
Visit Dhruva's Google Scholar page for a full list of publications.