Machine Learning
Machine Learning, if applied in the real world, needs to not only be accurate but also explainable and fair.”NOVI QUADRIANTO
Reader in Machine Learning
Machine learning is already involved in decision-making processes that affect peoples’ lives. Efficiency can be improved, costs can be reduced, and personalization of services and products can be greatly enhanced. However, concerns are rising about how to ensure that deployment of automated systems will follow clear, useful principles and requirements of trustworthiness. People should feel they can trust the systems they interact with, in terms of reliability of their predictions and decisions, capacity of the systems to understand their needs, and guarantees that they are genuinely driven by the goal of supporting them and not by the “secret” intention to favor some third party.
In the AI research group, we develop new artificial intelligence and machine learning models for auditing and mitigating inappropriate bias against protected subgroups, and improving transparency of algorithmic systems (“Ethical and Trustworthy AI”); for ensuring reliably good performance even when encountering extreme situations (“Safe and Robust AI”); and for facilitating a deep mutual understanding between a user and an algorithmic system “Active and Interactive AI”. We take diverse perspectives ranging from Bayesian approaches, structural causal models, AI cognitive foundations, to philosophy of AI.
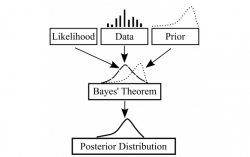
Algorithmic Fairness and Transparency
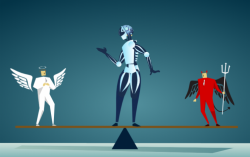
Reinforcement learning and active AI
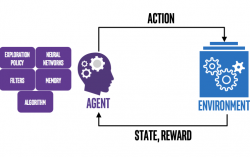
History and Philosophy of AI and Cognitive Science
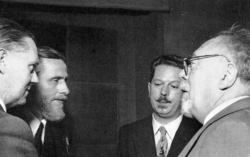