Key information
- Duration:
- 1 year full time, 2 years part time
- Start date:
- September 2024
- Apply by:
- 1 August 2024 (international), 1 September 2024 (UK)
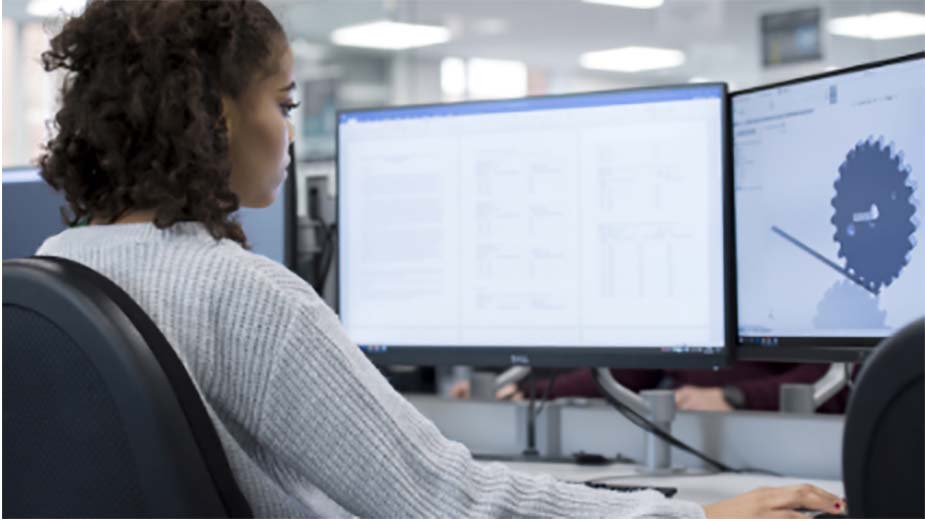
- 95% of our research overall in Mathematical Sciences was assessed to be world leading or internationally excellent (REF 2021)
Do you wish to work in industry or plan to pursue further study? Are you interested in data science and its application to science and technology? If so, this course is for you.
On this MSc, you’ll learn about the theory and practice of state-of-the-art data science with applications ranging from mathematics to physics, and from biology to computer science. You’ll develop the skills to provide and use appropriate and up-to-date tools to deal with large datasets common in industrial practice and academic science.
You’ll learn in an intellectually stimulating environment and develop your research skills. Your MSc dissertation, supported by one of our expert faculty, gives you the opportunity to explore in depth an area of your choice. You’ll be able to focus on the particular application area that interests you most.
We understand that deciding where and what to study is a very important decision. We’ll make all reasonable efforts to provide you with the courses, services and facilities described in this prospectus. However, if we need to make material changes, for example due to government or regulatory requirements, or unanticipated staff changes, we’ll let you know as soon as possible.
Most of my course is project based – great for my CV! I’ve already secured an internship doing machine learning. I believe my MSc can help me find my dream job!”Dong Wang
Data Science MSc
Data Science and Artificial Intelligence MSc webinar
Tuesday 7 May 2024, 10am–11am
Entry requirements
Degree requirements | You should normally have an upper second-class (2.1) undergraduate honours degree or above. |
---|---|
Subject-specific requirements | Your qualification should be in physics, engineering, science, computing, mathematics or life sciences. You may also be considered for the course if you have other professional qualifications or experience of equivalent standing. |
Please select your country from the list.
Argentina
Degree requirements | Licenciado/Titulo with a minimum final mark of at least 6.0-7.0 depending on your university. |
---|---|
Subject-specific requirements | Your qualification should be in physics, engineering, science, computing, mathematics or life sciences. You may also be considered for the course if you have other professional qualifications or experience of equivalent standing. |
Please note | Our entry requirements are guidelines and we assess all applications on a case-by-case basis. |
Australia
Degree requirements | Bachelors degree with a minimum second-class lower division. |
---|---|
Subject-specific requirements | Your qualification should be in physics, engineering, science, computing, mathematics or life sciences. You may also be considered for the course if you have other professional qualifications or experience of equivalent standing. |
Please note | Our entry requirements are guidelines and we assess all applications on a case-by-case basis. |
Azerbaijan
Degree requirements | Magistr or Specialist Diploma with a minimum average mark of at least 4 or 81% |
---|---|
Subject-specific requirements | Your qualification should be in physics, engineering, science, computing, mathematics or life sciences. You may also be considered for the course if you have other professional qualifications or experience of equivalent standing. |
Please note | Our entry requirements are guidelines and we assess all applications on a case-by-case basis. |
Bahrain
Degree requirements | Bachelors degree with a minimum CGPA 2.5/4.0 (Grade C+). |
---|---|
Subject-specific requirements | Your qualification should be in physics, engineering, science, computing, mathematics or life sciences. You may also be considered for the course if you have other professional qualifications or experience of equivalent standing. |
Please note | Our entry requirements are guidelines and we assess all applications on a case-by-case basis. |
Bangladesh
Degree requirements | A 4-year Bachelor degree with a minimum GPA of at least 3.2/4.0 |
---|---|
Subject-specific requirements | Your qualification should be in physics, engineering, science, computing, mathematics or life sciences. You may also be considered for the course if you have other professional qualifications or experience of equivalent standing. |
Please note | Our entry requirements are guidelines and we assess all applications on a case-by-case basis. |
Brazil
Degree requirements | Bacharel, Licenciado or professional title with a minimum final mark of at least 7. |
---|---|
Subject-specific requirements | Your qualification should be in physics, engineering, science, computing, mathematics or life sciences. You may also be considered for the course if you have other professional qualifications or experience of equivalent standing. |
Please note | Our entry requirements are guidelines and we assess all applications on a case-by-case basis. |
Brunei
Degree requirements | Bachelors (Honours) degree with a minimum second class lower division or CGPA 2.7/4.0. |
---|---|
Subject-specific requirements | Your qualification should be in physics, engineering, science, computing, mathematics or life sciences. You may also be considered for the course if you have other professional qualifications or experience of equivalent standing. |
Please note | Our entry requirements are guidelines and we assess all applications on a case-by-case basis. |
Canada
Degree requirements | Bachelors degree with a minimum CGPA 3.0/4.0 (grade B). |
---|---|
Subject-specific requirements | Your qualification should be in physics, engineering, science, computing, mathematics or life sciences. You may also be considered for the course if you have other professional qualifications or experience of equivalent standing. |
Please note | Our entry requirements are guidelines and we assess all applications on a case-by-case basis. |
Chile
Degree requirements | Licenciado with a minimum final mark of at least 4.5-5.0/7 depending on your university. |
---|---|
Subject-specific requirements | Your qualification should be in physics, engineering, science, computing, mathematics or life sciences. You may also be considered for the course if you have other professional qualifications or experience of equivalent standing. |
Please note | Our entry requirements are guidelines and we assess all applications on a case-by-case basis. |
China
Degree requirements | Bachelors degree with a minimum overall mark of at least 70% - 80% depending on your university. Sussex uses the Shanghai Academic Ranking of World Universities to determine offer levels. See: www.shanghairanking.com/rankings/2021 As evidence of completing your degree you must provide both a Degree Certificate and Graduation Certificate. |
---|---|
Subject-specific requirements | Your qualification should be in physics, engineering, science, computing, mathematics or life sciences. You may also be considered for the course if you have other professional qualifications or experience of equivalent standing. |
Please note | Our entry requirements are guidelines and we assess all applications on a case-by-case basis. |
Colombia
Degree requirements | Licenciado with ‘Acreditacion de alta calidad’ and a minimum CGPA of 3.0. |
---|---|
Subject-specific requirements | Your qualification should be in physics, engineering, science, computing, mathematics or life sciences. You may also be considered for the course if you have other professional qualifications or experience of equivalent standing. |
Please note | Our entry requirements are guidelines and we assess all applications on a case-by-case basis. |
Cyprus
Degree requirements | Bachelors degree or Ptychion with a minimum final mark of at least 6.5. |
---|---|
Subject-specific requirements | Your qualification should be in physics, engineering, science, computing, mathematics or life sciences. You may also be considered for the course if you have other professional qualifications or experience of equivalent standing. |
Please note | Our entry requirements are guidelines and we assess all applications on a case-by-case basis. |
Denmark
Degree requirements | Bachelors degree with a minimum overall mark of at least 7 (Fair Performance). |
---|---|
Subject-specific requirements | Your qualification should be in physics, engineering, science, computing, mathematics or life sciences. You may also be considered for the course if you have other professional qualifications or experience of equivalent standing. |
Please note | Our entry requirements are guidelines and we assess all applications on a case-by-case basis. |
Ecuador
Degree requirements | Licenciado with a minimum final mark of at least 15/20. |
---|---|
Subject-specific requirements | Your qualification should be in physics, engineering, science, computing, mathematics or life sciences. You may also be considered for the course if you have other professional qualifications or experience of equivalent standing. |
Please note | Our entry requirements are guidelines and we assess all applications on a case-by-case basis. |
Egypt
Degree requirements | Bachelors degree from a university with a minimum overall grade of at least 70%. |
---|---|
Subject-specific requirements | Your qualification should be in physics, engineering, science, computing, mathematics or life sciences. You may also be considered for the course if you have other professional qualifications or experience of equivalent standing. |
Please note | Our entry requirements are guidelines and we assess all applications on a case-by-case basis. |
France
Degree requirements | Licence with mention assez bien or Maîtrise with a minimum final mark of at least 12. |
---|---|
Subject-specific requirements | Your qualification should be in physics, engineering, science, computing, mathematics or life sciences. You may also be considered for the course if you have other professional qualifications or experience of equivalent standing. |
Please note | Our entry requirements are guidelines and we assess all applications on a case-by-case basis. |
Germany
Degree requirements | Bachelors degree or Magister Artium with a minimum final mark of 2.7 or better. |
---|---|
Subject-specific requirements | Your qualification should be in physics, engineering, science, computing, mathematics or life sciences. You may also be considered for the course if you have other professional qualifications or experience of equivalent standing. |
Please note | Our entry requirements are guidelines and we assess all applications on a case-by-case basis. |
Ghana
Degree requirements | Bachelors degree from a public university with a minimum second-class lower division. |
---|---|
Subject-specific requirements | Your qualification should be in physics, engineering, science, computing, mathematics or life sciences. You may also be considered for the course if you have other professional qualifications or experience of equivalent standing. |
Please note | Our entry requirements are guidelines and we assess all applications on a case-by-case basis. |
Greece
Degree requirements | Ptychion from an AEI with a minimum final mark of at least 6.5. |
---|---|
Subject-specific requirements | Your qualification should be in physics, engineering, science, computing, mathematics or life sciences. You may also be considered for the course if you have other professional qualifications or experience of equivalent standing. |
Please note | Our entry requirements are guidelines and we assess all applications on a case-by-case basis. |
Hong Kong
Degree requirements | Bachelors (Honours) degree with a minimum second-class lower division. |
---|---|
Subject-specific requirements | Your qualification should be in physics, engineering, science, computing, mathematics or life sciences. You may also be considered for the course if you have other professional qualifications or experience of equivalent standing. |
Please note | Our entry requirements are guidelines and we assess all applications on a case-by-case basis. |
India
Degree requirements | Bachelors degree with a minimum overall mark of at least 50-65% depending on your university. |
---|---|
Subject-specific requirements | Your qualification should be in physics, engineering, science, computing, mathematics or life sciences. You may also be considered for the course if you have other professional qualifications or experience of equivalent standing. |
Please note | Our entry requirements are guidelines and we assess all applications on a case-by-case basis. |
Indonesia
Degree requirements | Bachelors degree from an 'A' accredited university with a minimum CGPA of 2.8/4.0. Bachelors degree from a 'B' accredited university with a minimum CGPA of 3.0/4.0. |
---|---|
Subject-specific requirements | Your qualification should be in physics, engineering, science, computing, mathematics or life sciences. You may also be considered for the course if you have other professional qualifications or experience of equivalent standing. |
Please note | Our entry requirements are guidelines and we assess all applications on a case-by-case basis. |
Iran
Degree requirements | Bachelors degree (Licence or Karshenasi) with a minimum final mark of at least 14. |
---|---|
Subject-specific requirements | Your qualification should be in physics, engineering, science, computing, mathematics or life sciences. You may also be considered for the course if you have other professional qualifications or experience of equivalent standing. |
Please note | Our entry requirements are guidelines and we assess all applications on a case-by-case basis. |
Italy
Degree requirements | Diploma di Laurea with a minimum overall mark of at least 101. |
---|---|
Subject-specific requirements | Your qualification should be in physics, engineering, science, computing, mathematics or life sciences. You may also be considered for the course if you have other professional qualifications or experience of equivalent standing. |
Please note | Our entry requirements are guidelines and we assess all applications on a case-by-case basis. |
Japan
Degree requirements | Bachelors degree with a minimum C/GPA of at least 3.0/4.0 or equivalent. |
---|---|
Subject-specific requirements | Your qualification should be in physics, engineering, science, computing, mathematics or life sciences. You may also be considered for the course if you have other professional qualifications or experience of equivalent standing. |
Please note | Our entry requirements are guidelines and we assess all applications on a case-by-case basis. |
Jordan
Degree requirements | Bachelors degree with a minimum CGPA of at least 2.8/4.0 or 75%. |
---|---|
Subject-specific requirements | Your qualification should be in physics, engineering, science, computing, mathematics or life sciences. You may also be considered for the course if you have other professional qualifications or experience of equivalent standing. |
Please note | Our entry requirements are guidelines and we assess all applications on a case-by-case basis. |
Kazakhstan
Degree requirements | Bachelors degree with a minimum overall mark of 3.75 or better (on a scale of 1-5)/CGPA 2,67. |
---|---|
Subject-specific requirements | Your qualification should be in physics, engineering, science, computing, mathematics or life sciences. You may also be considered for the course if you have other professional qualifications or experience of equivalent standing. |
Please note | Our entry requirements are guidelines and we assess all applications on a case-by-case basis. |
Kenya
Degree requirements | Bachelors (Honours) degree with a minimum second-class lower division. |
---|---|
Subject-specific requirements | Your qualification should be in physics, engineering, science, computing, mathematics or life sciences. You may also be considered for the course if you have other professional qualifications or experience of equivalent standing. |
Please note | Our entry requirements are guidelines and we assess all applications on a case-by-case basis. |
Kuwait
Degree requirements | Bachelors degree with a minimum CGPA of at least 2.8/4.0 or B. |
---|---|
Subject-specific requirements | Your qualification should be in physics, engineering, science, computing, mathematics or life sciences. You may also be considered for the course if you have other professional qualifications or experience of equivalent standing. |
Please note | Our entry requirements are guidelines and we assess all applications on a case-by-case basis. |
Lebanon
Degree requirements | Bachelors degree with a minimum CGPA 3.0/4.0 or 13/20. |
---|---|
Subject-specific requirements | Your qualification should be in physics, engineering, science, computing, mathematics or life sciences. You may also be considered for the course if you have other professional qualifications or experience of equivalent standing. |
Please note | Our entry requirements are guidelines and we assess all applications on a case-by-case basis. |
Malawi
Degree requirements | Masters degree, depending on your university. |
---|---|
Subject-specific requirements | Your qualification should be in physics, engineering, science, computing, mathematics or life sciences. You may also be considered for the course if you have other professional qualifications or experience of equivalent standing. |
Please note | Our entry requirements are guidelines and we assess all applications on a case-by-case basis. |
Malaysia
Degree requirements | Bachelors degree with a minimum CGPA of at least 2.7/4.0. |
---|---|
Subject-specific requirements | Your qualification should be in physics, engineering, science, computing, mathematics or life sciences. You may also be considered for the course if you have other professional qualifications or experience of equivalent standing. |
Please note | Our entry requirements are guidelines and we assess all applications on a case-by-case basis. |
Mexico
Degree requirements | Licenciado with a minimum final mark of at least 7/10. |
---|---|
Subject-specific requirements | Your qualification should be in physics, engineering, science, computing, mathematics or life sciences. You may also be considered for the course if you have other professional qualifications or experience of equivalent standing. |
Please note | Our entry requirements are guidelines and we assess all applications on a case-by-case basis. |
Nepal
Degree requirements | A 4-year Bachelor degree with a minimum GPA of at least 3.0/4.0 |
---|---|
Subject-specific requirements | Your qualification should be in physics, engineering, science, computing, mathematics or life sciences. You may also be considered for the course if you have other professional qualifications or experience of equivalent standing. |
Please note | Our entry requirements are guidelines and we assess all applications on a case-by-case basis. |
Nigeria
Degree requirements | Bachelors degree with a minimum second-class lower division or CGPA of at least 2.8-2.99/4.0, 3.0-3.49/5.0, or 4.0-4.6/7.0. |
---|---|
Subject-specific requirements | Your qualification should be in physics, engineering, science, computing, mathematics or life sciences. You may also be considered for the course if you have other professional qualifications or experience of equivalent standing. |
Please note | Our entry requirements are guidelines and we assess all applications on a case-by-case basis. |
Norway
Degree requirements | Bachelors degree with a minimum overall grade of C. |
---|---|
Subject-specific requirements | Your qualification should be in physics, engineering, science, computing, mathematics or life sciences. You may also be considered for the course if you have other professional qualifications or experience of equivalent standing. |
Please note | Our entry requirements are guidelines and we assess all applications on a case-by-case basis. |
Oman
Degree requirements | Bachelors degree with a minimum CGPA of at least 2.8/4.0. |
---|---|
Subject-specific requirements | Your qualification should be in physics, engineering, science, computing, mathematics or life sciences. You may also be considered for the course if you have other professional qualifications or experience of equivalent standing. |
Please note | Our entry requirements are guidelines and we assess all applications on a case-by-case basis. |
Pakistan
Degree requirements | Four-year bachelors degree with a minimum overall grade of 60% or Masters with a minimum 55%. |
---|---|
Subject-specific requirements | Your qualification should be in physics, engineering, science, computing, mathematics or life sciences. You may also be considered for the course if you have other professional qualifications or experience of equivalent standing. |
Please note | Our entry requirements are guidelines and we assess all applications on a case-by-case basis. |
Palestine
Degree requirements | Bachelors degree with a minimum of at least 70% or CGPA of at least 3.0/4.0 |
---|---|
Subject-specific requirements | Your qualification should be in physics, engineering, science, computing, mathematics or life sciences. You may also be considered for the course if you have other professional qualifications or experience of equivalent standing. |
Please note | Our entry requirements are guidelines and we assess all applications on a case-by-case basis. |
Paraguay
Degree requirements | Bachelors with a minimum final mark of at least 7/10. |
---|---|
Subject-specific requirements | Your qualification should be in physics, engineering, science, computing, mathematics or life sciences. You may also be considered for the course if you have other professional qualifications or experience of equivalent standing. |
Please note | Our entry requirements are guidelines and we assess all applications on a case-by-case basis. |
Peru
Degree requirements | Licenciado with a minimum final mark of at least 12/20 from a public university or 14/20 from a private university. |
---|---|
Subject-specific requirements | Your qualification should be in physics, engineering, science, computing, mathematics or life sciences. You may also be considered for the course if you have other professional qualifications or experience of equivalent standing. |
Please note | Our entry requirements are guidelines and we assess all applications on a case-by-case basis. |
Philippines
Degree requirements | Masters degree with a minimum of 1.75/5.0 (where 1 is the highest) or 3.5/4.0 |
---|---|
Subject-specific requirements | Your qualification should be in physics, engineering, science, computing, mathematics or life sciences. You may also be considered for the course if you have other professional qualifications or experience of equivalent standing. |
Please note | Our entry requirements are guidelines and we assess all applications on a case-by-case basis. |
Qatar
Degree requirements | Bachelors degree with a minimum overall CPGA of at least 2.8 (on a scale of 4). |
---|---|
Subject-specific requirements | Your qualification should be in physics, engineering, science, computing, mathematics or life sciences. You may also be considered for the course if you have other professional qualifications or experience of equivalent standing. |
Please note | Our entry requirements are guidelines and we assess all applications on a case-by-case basis. |
Russia
Degree requirements | Bakalavr or Specialist Diploma with a minimum average mark of at least 4. |
---|---|
Subject-specific requirements | Your qualification should be in physics, engineering, science, computing, mathematics or life sciences. You may also be considered for the course if you have other professional qualifications or experience of equivalent standing. |
Please note | Our entry requirements are guidelines and we assess all applications on a case-by-case basis. |
Saudi Arabia
Degree requirements | Bachelors degree with a minimum CGPA of 3.0/5.0 or 2.8/4.0. |
---|---|
Subject-specific requirements | Your qualification should be in physics, engineering, science, computing, mathematics or life sciences. You may also be considered for the course if you have other professional qualifications or experience of equivalent standing. |
Please note | Our entry requirements are guidelines and we assess all applications on a case-by-case basis. |
Singapore
Degree requirements | Bachelors (Honours) degree with a minimum second-class lower division or CAP 3.5. |
---|---|
Subject-specific requirements | Your qualification should be in physics, engineering, science, computing, mathematics or life sciences. You may also be considered for the course if you have other professional qualifications or experience of equivalent standing. |
Please note | Our entry requirements are guidelines and we assess all applications on a case-by-case basis. |
South Africa
Degree requirements | Bachelors (honours) degree with a minimum second-class division 2. |
---|---|
Subject-specific requirements | Your qualification should be in physics, engineering, science, computing, mathematics or life sciences. You may also be considered for the course if you have other professional qualifications or experience of equivalent standing. |
Please note | Our entry requirements are guidelines and we assess all applications on a case-by-case basis. |
South Korea
Degree requirements | Bachelors degree with a minimum CGPA of at least 3.0/4.5 or 2.8/4.3 or B. |
---|---|
Subject-specific requirements | Your qualification should be in physics, engineering, science, computing, mathematics or life sciences. You may also be considered for the course if you have other professional qualifications or experience of equivalent standing. |
Please note | Our entry requirements are guidelines and we assess all applications on a case-by-case basis. |
Spain
Degree requirements | Licenciado with a minimum final mark of at least 2/4 or 6/10. |
---|---|
Subject-specific requirements | Your qualification should be in physics, engineering, science, computing, mathematics or life sciences. You may also be considered for the course if you have other professional qualifications or experience of equivalent standing. |
Please note | Our entry requirements are guidelines and we assess all applications on a case-by-case basis. |
Sri Lanka
Degree requirements | Bachelors Special degree with a minimum lower second honours. |
---|---|
Subject-specific requirements | Your qualification should be in physics, engineering, science, computing, mathematics or life sciences. You may also be considered for the course if you have other professional qualifications or experience of equivalent standing. |
Please note | Our entry requirements are guidelines and we assess all applications on a case-by-case basis. |
Switzerland
Degree requirements | Licence or Diplôme with a minimum 4.5/6 or 7/10. |
---|---|
Subject-specific requirements | Your qualification should be in physics, engineering, science, computing, mathematics or life sciences. You may also be considered for the course if you have other professional qualifications or experience of equivalent standing. |
Please note | Our entry requirements are guidelines and we assess all applications on a case-by-case basis. |
Taiwan
Degree requirements | Bachelors degree with a minimum overall mark of at least 65%-75% depending on your university. |
---|---|
Subject-specific requirements | Your qualification should be in physics, engineering, science, computing, mathematics or life sciences. You may also be considered for the course if you have other professional qualifications or experience of equivalent standing. |
Please note | Our entry requirements are guidelines and we assess all applications on a case-by-case basis. |
Thailand
Degree requirements | Bachelors degree with a minimum CGPA of at least 2.5 -2.8/4.0 or equivalent depending on your university. |
---|---|
Subject-specific requirements | Your qualification should be in physics, engineering, science, computing, mathematics or life sciences. You may also be considered for the course if you have other professional qualifications or experience of equivalent standing. |
Please note | Our entry requirements are guidelines and we assess all applications on a case-by-case basis. |
Turkey
Degree requirements | Lisans Diplomasi with a minimum CGPA of at least 2.6 - 2.8/4.0 or equivalent depending on your university. |
---|---|
Subject-specific requirements | Your qualification should be in physics, engineering, science, computing, mathematics or life sciences. You may also be considered for the course if you have other professional qualifications or experience of equivalent standing. |
Please note | Our entry requirements are guidelines and we assess all applications on a case-by-case basis. |
United Arab Emirates
Degree requirements | Bachelors degree with a minimum CGPA of at least 2.8/4.0 or equivalent. |
---|---|
Subject-specific requirements | Your qualification should be in physics, engineering, science, computing, mathematics or life sciences. You may also be considered for the course if you have other professional qualifications or experience of equivalent standing. |
Please note | Our entry requirements are guidelines and we assess all applications on a case-by-case basis. |
USA
Degree requirements | Bachelors degree with a minimum CGPA of at least 3.0/4.0. |
---|---|
Subject-specific requirements | Your qualification should be in physics, engineering, science, computing, mathematics or life sciences. You may also be considered for the course if you have other professional qualifications or experience of equivalent standing. |
Please note | Our entry requirements are guidelines and we assess all applications on a case-by-case basis. |
Vietnam
Degree requirements | Bachelors degree (with a Graduate Thesis/research component) with a minimum CGPA of at least 3.0/4.0 or 7.0/10. As evidence of completing your degree you must provide both proof of graduation in addition to your transcript. |
---|---|
Subject-specific requirements | Your qualification should be in physics, engineering, science, computing, mathematics or life sciences. You may also be considered for the course if you have other professional qualifications or experience of equivalent standing. |
Please note | Our entry requirements are guidelines and we assess all applications on a case-by-case basis. |
Zambia
Degree requirements | Masters degree with a minimum GPA of 2.0/2.5 or equivalent. |
---|---|
Subject-specific requirements | Your qualification should be in physics, engineering, science, computing, mathematics or life sciences. You may also be considered for the course if you have other professional qualifications or experience of equivalent standing. |
Please note | Our entry requirements are guidelines and we assess all applications on a case-by-case basis. |
Zimbabwe
Degree requirements | Bachelors (Honours) degree with a minimum second-class lower division. |
---|---|
Subject-specific requirements | Your qualification should be in physics, engineering, science, computing, mathematics or life sciences. You may also be considered for the course if you have other professional qualifications or experience of equivalent standing. |
Please note | Our entry requirements are guidelines and we assess all applications on a case-by-case basis. |
My country is not listed
If your country is not listed, you need to contact us and find out the qualification level you should have for this course. Contact us
Subject-specific requirements | Your qualification should be in physics, engineering, science, computing, mathematics or life sciences. You may also be considered for the course if you have other professional qualifications or experience of equivalent standing. |
---|
English language requirements
IELTS (Academic)
Standard level (6.0 overall, including at least 5.5 in each component).
IELTS scores are valid for two years from the test date. You cannot combine scores from more than one sitting of the test. Your score must be valid when you begin your Sussex course. Find out more about IELTS
We accept IELTS One Skills Retake.
We do not accept IELTS Online.
Alternative English language qualifications
Proficiency tests
Cambridge Advanced Certificate in English (CAE)
169 overall, including at least 162 in each skill.
We would normally expect the CAE test to have been taken within two years before the start of your course.
You cannot combine scores from more than one sitting of the test. Find out more about Cambridge English: Advanced
Cambridge Certificate of Proficiency in English (CPE)
169 overall, including at least 162 in each skill.
We would normally expect the CPE test to have been taken within two years before the start of your course.
You cannot combine scores from more than one sitting of the test. Find out more about Cambridge English: Proficiency
LanguageCert International ESOL SELT
International ESOL SELT B2 with a minimum of 36 in each component.
LanguageCert International ESOL scores are valid for two years from the test date. Your score must be valid when you begin your Sussex course. Find out more about LanguageCert SELT
We only accept LanguageCert when taken at SELT Test Centres. We do not accept the online version.
Pearson (PTE Academic)
59 overall, including at least 59 in all four skills.
PTE (Academic) scores are valid for two years from the test date. You cannot combine scores from more than one sitting of the test. Your score must be valid when you begin your Sussex course. Find out more about Pearson (PTE Academic)
We do not accept the PTE Academic Online test.
TOEFL (iBT)
80 overall, including at least 17 in Listening, 18 in Reading, 20 in Speaking, 17 in Writing.
TOEFL (iBT) scores are valid for two years from the test date. You cannot combine scores from more than one sitting of the test. Your score must be valid when you begin your Sussex course. Find out more about TOEFL (iBT)
We do not accept TOEFL (iBT) Home Edition.
The TOEFL Institution Code for the University of Sussex is 9166.
English language qualifications
AS/A-level (GCE)
Grade C or above in English Language.
Hong Kong Advanced Level Examination (HKALE)/ AS or A Level: grade C or above in Use of English.
GCE O-level
Grade C or above in English.
Brunei/Cambridge GCE O-level in English: grades 1-6.
Singapore/Cambridge GCE O-level in English: grades 1-6.
GCSE or IGCSE
Grade C or above in English as a First Language (Grade 4 or above in GCSE from 2017).
Grade B or above in English as a Second Language.
Ghana Senior Secondary School Certificate
If awarded before 1993: grades 1-6 in English language.
If awarded between 1993 and 2005: grades A-D in English language.
Hong Kong Diploma of Secondary Education (HKDSE)
Level 4, including at least 3 in each component in English Language.
Indian School Certificate (Standard XII)
The Indian School Certificate is accepted at the grades below when awarded by the following examination boards:
Central Board of Secondary Education (CBSE) – English Core only: 70%
Council for Indian School Certificate Examinations (CISCE) - English: 70%
International Baccalaureate Diploma (IB)
English A or English B at grade 5 or above.
Kenya Certificate of Secondary Education
Grades A - C in English language
Malaysian Certificate of Education (SPM) 1119/GCE O-level
If taken before the end of 2008: grades 1-6 in English Language.
If taken from 2009 onwards: grade C or above in English Language.
The qualification must be jointly awarded by the University of Cambridge Local Examinations Syndicate (UCLES).
West African Senior School Certificate
Grades A1-C6 (1-6) in English language when awarded by the West African Examinations Council (WAEC) or the National Examinations Council (NECO).
Country exceptions
Select to see the list of exempt English-speaking countries
If you are a national of one of the countries below, or if you have recently completed a qualification equivalent to a UK Bachelors degree or higher in one of these countries, you will normally meet our English requirement. Note that qualifications obtained by distance learning or awarded by studying outside these countries cannot be accepted for English language purposes.
You will normally be expected to have completed the qualification within two years before starting your course at Sussex. If the qualification was obtained earlier than this, we would expect you to be able to demonstrate that you have maintained a good level of English, for example by living in an English-speaking country or working in an occupation that required you to use English regularly and to a high level.
Please note that this list is determined by the UK’s Home Office, not by the University of Sussex.
List of exempt countries:
- Antigua and Barbuda
- Australia
- Bahamas
- Barbados
- Belize
- Canada**
- Dominica
- Grenada
- Guyana
- Ireland
- Jamaica
- New Zealand
- St Kitts and Nevis
- St Lucia
- St Vincent and the Grenadines
- The British Overseas Territories
- Trinidad and Tobago
- United Kingdom
- USA
** Canada: you must be a national of Canada; other nationals not on this list who have a degree from a Canadian institution will not normally be exempt from needing to provide evidence of English.
English language support
If you don’t meet the English language requirements for your degree, you may be able to take a pre-sessional course
Visas and immigration
Find out how to apply for a student visa
Admissions information for applicants
How to apply | You apply to Sussex using our postgraduate application system
|
---|---|
Personal statement | Yes. You must submit a personal statement as part of your application. |
If your qualifications aren’t listed or you have a question about entry requirements, contact us
For details on any additional costs, check out the Fees and scholarships section.
Application deadlines
1 August 2024 (international), 1 September 2024 (UK)
We strongly recommend an earlier application where possible, as some courses are in high demand and may close before the above dates. Find out more at How to apply for a Masters course
Modules
Full-time and part-time study
Choose to study this course full time or part time, to fit around your work and personal life. Modules for the full-time course are listed below. For details about the part-time course, contact us.
Core modules
Core modules are taken by all students on the course. They give you a solid grounding in your chosen subject and prepare you to explore the topics that interest you most.
All year
Autumn teaching
Spring teaching
Options
Alongside your core modules, you can choose options to broaden your horizons and tailor your course to your interests. This list gives you a flavour of our options, which are kept under review and may change, for example in response to student feedback or the latest research.
While it’s our aim for students to take their preferred combinations of options, this can’t be guaranteed and will be subject to timetabling. Options may be grouped and if so, students will be able to choose a set number of options from the selection available in any particular group.
Autumn teaching
- Advanced Methods in Molecular Research
- Advanced Numerical Analysis (L.7)
- Applied Natural Language Processing
- Data Analysis Techniques
- Linear Statistical Models (L7)
- Mathematics and Computational Methods for Complex Systems
- Probability Models (L7)
- Programming through Python
Spring teaching
- Advanced Natural Language Processing
- Frontiers in Particle Physics
- Genomics and Bioinformatics
- Image Processing
- Machine Learning and Statistics for Health (L7)
- Monte Carlo Simulations (L7)
- Numerical Solution of Partial Differential Equations (L.7)
- Particle Physics Detector Technology
- Statistical Inference (L.7)
- Wearable Technologies
- Web Applications and Services
We regularly review our modules to incorporate student feedback, staff expertise, as well as the latest research and teaching methodology. We’re planning to run these modules in the academic year 2024/25. However, there may be changes to these modules in response to feedback, staff availability, student demand or updates to our curriculum. We’ll make sure to let you know of any material changes to modules at the earliest opportunity.
We’ll do our best to provide as much optional choice as we can, but timetabling constraints mean it may not be possible to take some module combinations. The structure of a small number of courses means that the order of modules or the streams you choose may determine whether modules are core or optional. This means that your core modules or options may differ from what’s shown here.
Our experts
Rapid advances in artificial intelligence, computing, mathematics and statistics are empowering scientists and engineers, and changing the way they operate. Join us and be part of this revolution!”Dr Omar Lakkis
Reader in Mathematics and Convener of the Data Science MSc
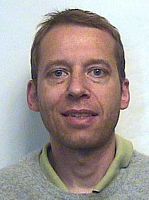
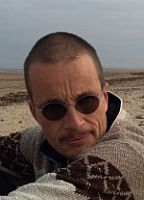
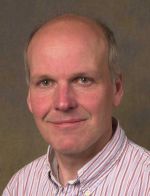
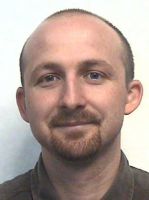
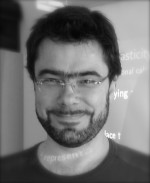
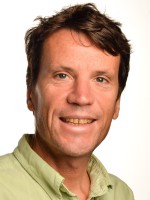
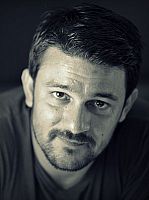
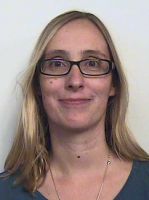
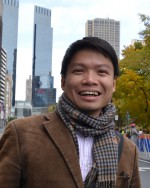
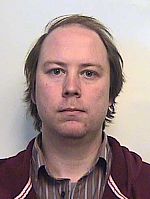
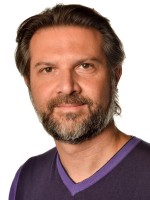
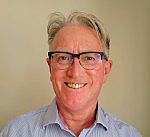
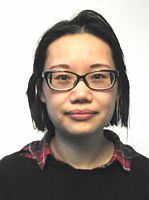
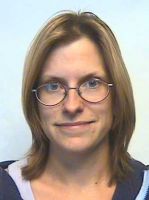
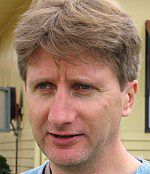
Fees and scholarships
How much does it cost?
Fees
- Home students:
- £10,500 per year for full-time students
- Channel Islands and Isle of Man students:
- £10,500 per year for full-time students
- International students:
- £21,500 per year for full-time students
If you study part time over two years, you’ll be charged 50% of the equivalent full-time fee in each year of study. Your second-year fee – if you continue your studies without a break – will be subject to a 3% increase (subject to rounding).
If you’re a self-funded international student, you’re required to pay a tuition fee deposit. Find out more about Masters tuition fee deposits
Living costs
Find out typical living costs for studying at Sussex
Find out about our terms and conditions
How can I fund my course?
Scholarships
Our goal is to ensure that every student who wants to study with us is able to regardless of financial barriers, so that we continue to attract talented and unique people.
-
Artificial Intelligence and Data Science Postgraduate Conversion Scholarship
£160,000 of scholarships available to people from groups currently underrepresented in the fields of AI and Data Science
-
Aziz Foundation Scholarship
A full fee waiver for British Muslim Masters applicants
-
Chancellor's International Scholarships
Scholarships worth £5,000 available to international applicants
-
Chancellor's Masters Scholarship
100 scholarships worth up to £5,000 are available for First Class graduates from UK or Irish universities
-
Climate Leaders Scholarship
£3,000 scholarships available to environmental influencers bringing about real-world behaviour change
-
Dr John Birch Music Scholarship (Organ)
£800 scholarship available to reward talented organ player studying on any course at Sussex.
-
Dr John Birch Music Scholarships
Scholarships of £800 are available to reward talented musicians studying on any course at Sussex
-
Friends of the University of Sussex Scholarship
$10,000 USD scholarship for a national or citizen of the USA
-
HESPAL (Higher Education Scholarships Scheme for the Palestinian Territories)
One full tuition fee waiver in conjunction with maintenance support from the British Council are available to a resident of Palestinian Territories
-
Jenny and Peter Kingsland Scholarship
£5,000 scholarship for women in STEM
-
Mandela Scholarship
Full fee waiver and maintenance award for an applicant from South Africa
-
Saïd Foundation Scholarships
5 full scholarships available for Masters students from Jordan, Lebanon, Palestine and Syria
-
Sanctuary Scholarship
Fully funded scholarships available to support a forced migrant seeking to study a Masters at Sussex
-
Sports Scholarships Scheme
Cash scholarships available for students who have demonstrated sporting excellence
-
Sussex Alumni Award
£2,000 award for previous Sussex graduates returning to study a Masters degree
-
Sussex Bangladesh Scholarship
Unlimited £4,000 scholarships for applicants who are nationals of Bangladesh
-
Sussex Egypt Scholarship
Unlimited £4,000 scholarships for applicants who are nationals of Egypt
-
Sussex Graduate Scholarship
Scholarships up to £4,000 for 2024 Sussex graduates progressing directly to a Masters at Sussex
-
Sussex GREAT Scholarship China
£10,000 scholarship for an applicant from China
-
Sussex GREAT Scholarship Indonesia
£10,000 scholarship for an applicant from Indonesia
-
Sussex GREAT Scholarship Mexico
£10,000 scholarship for an applicant from Mexico
-
Sussex India Scholarship
Unlimited £4,000 scholarships for applicants who are nationals of India
-
Sussex Nigeria Scholarship
Unlimited £4,000 scholarships for applicants who are nationals of Nigeria
-
Sussex Pakistan Scholarship
Unlimited £4,000 scholarships for applicants who are nationals of Pakistan
-
Sussex Turkey Scholarship
Unlimited £4,000 scholarships for applicants who are nationals of Turkey
-
Sussex Vietnam Scholarship
Unlimited £4,000 scholarships for applicants who are nationals of Vietnam
-
Technopolis UK Masters Scholarship
Two £14,000 Scholarships for Black British students in the University of Sussex Business School and the School of Mathematical and Physical Sciences
Working while you study
Our Careers and Employability Centre can help you find part-time work while you study. Find out more about career development and part-time work
Careers
There’s strong employer demand for skilled people in artificial intelligence and data science. The UK Government’s Digital Strategy predicts that within 20 years, 90% of all jobs will require some element of digital skills.
The versatility of this course is highlighted by a wide range of career options. Typically, our graduates become data scientists in industry, particularly in:
- technology and engineering
- computing
- finance and banking.
Other careers paths may include:
- management
- Civil Service
- scientific research
- teaching
- academia.