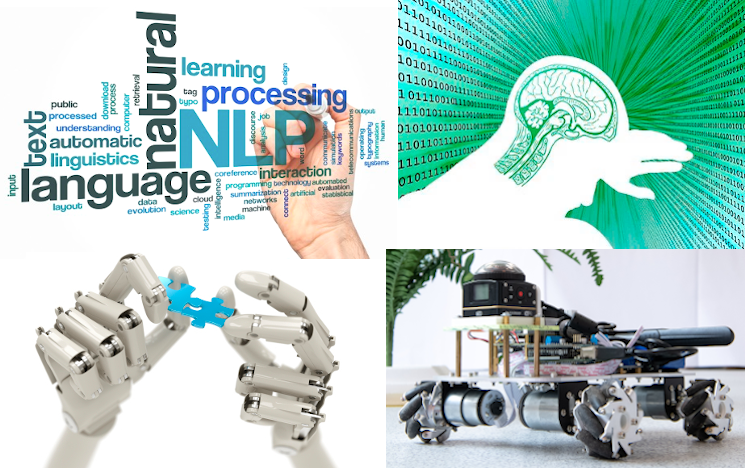
AI Research Group
The AI Research Group at the University of Sussex was created through the merger of the Evolutionary and Adaptive Systems (EASy) and the Data Science groups. We conduct research and teach a wide range of AI related topics, often cross-disciplinary and with a unique Sussex angle. We collaborate internationally and locally with academics, businesses and public stakeholders, working at the frontiers of knowledge, on solving real-world problems, and in policy and public outreach.
Sussex AI: A Centre of Excellence
Sussex AI is an interdisciplinary Centre of Excellence at the University of Sussex and the focal point of research in Artificial Intelligence methods and applications. Our centre draws together world-leading experts from across the University to create a critical mass of technical skills and domain knowledge. Our research and training covers a wide range of topics across AI and data science, with a unique multidisciplinary Sussex angle.
Sussex AI is directed by Prof. Thomas Nowotny and Prof. Julie Weeds based in the AI Research Group, more details below.
A new website for Sussex AI will be available in the coming weeks. Follow us on LinkedIn for up to date information.
Research Centres
Members of the AI research group direct or co-direct a number of cross-campus interdisciplinary research centres through which a considerable part of the group's research is conducted.
Prof Nowotny and Prof Philippides co-direct The Centre for Computational Neuroscience and Robotics (CCNR) jointly with Prof Graham in the School of Life Sciences. The CCNR focuses on aspects of computational neuroscience and computational biology, along with research on bio-inspired adaptive robotics, bio-inspired adaptive computing and complex adaptive systems.
Dr Chrisley directs the The Centre for Research in Cognitive Science (COGS) which fosters interaction and collaboration among all those working in cognitive science at Sussex, including researchers and students in artificial intelligence, psychology, linguistics, neuroscience and philosophy.
Prof Seth co-directs The Sussex Centre for Consciousness Science with Prof Critchley in the Brighton and Sussex Medical School. The SCCS focuses on basic science that seeks to unravel the complex brain mechanisms that generate consciousness, along with research that translates insights about the mechanisms of consciousness to the clinical domain.
Dr Weeds co-directs The Data Intensive Science Center, University of Sussex (DISCUS) with Prof Oliver in the School of Mathematics and Physics. DISCUS is concerned with the application of modern Data Science and Machine Learning to real world problems and research in other disciplines.
Dr Buckley is a co-director of The Sussex Centre for Sensory Neuroscience and Computing (SNAC) along side Prof Baden and other colleagues in Life Sciences and Psychology. SNAC brings together research labs in three schools by their research interest in the senses and their computational underpinnings.
Other relevant research centres the AI group is deeply involved in include The Sussex Humanities Lab (SHL) and Sussex Neuroscience.
Latest news
Contact
Head of the AI Research Group is Professor Thomas Nowotny.