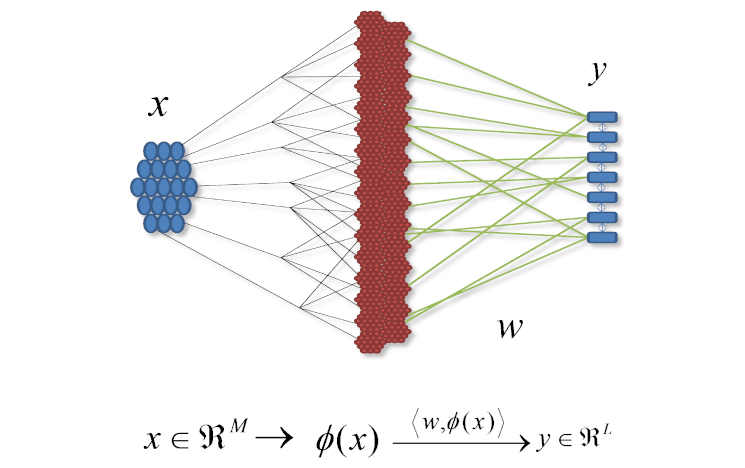
Research
Our research is cross-disciplinary, involving a large network of leading fellow scientists at Sussex, and many collaborators both in the UK and worldwide. Read on below to find out more about our research topics and the people working on them.
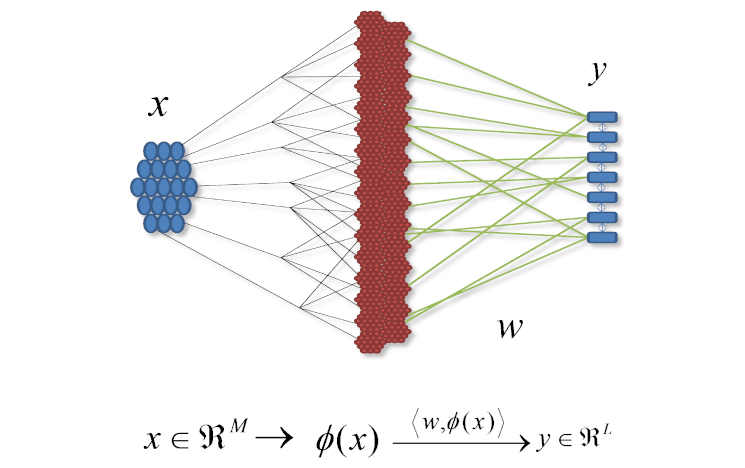
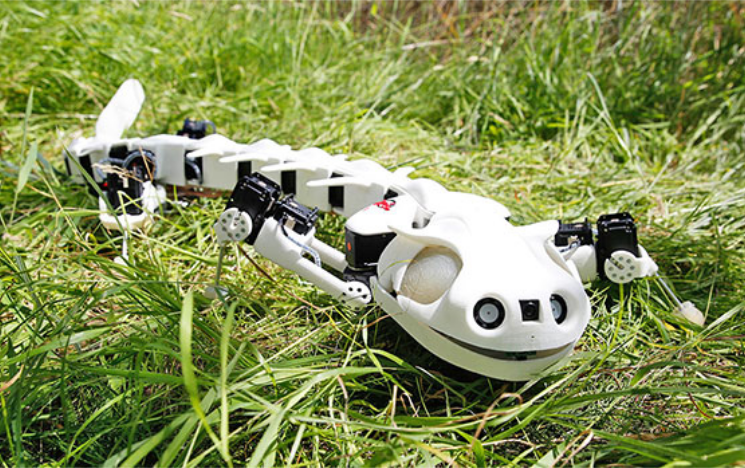
Bio-inspired AI
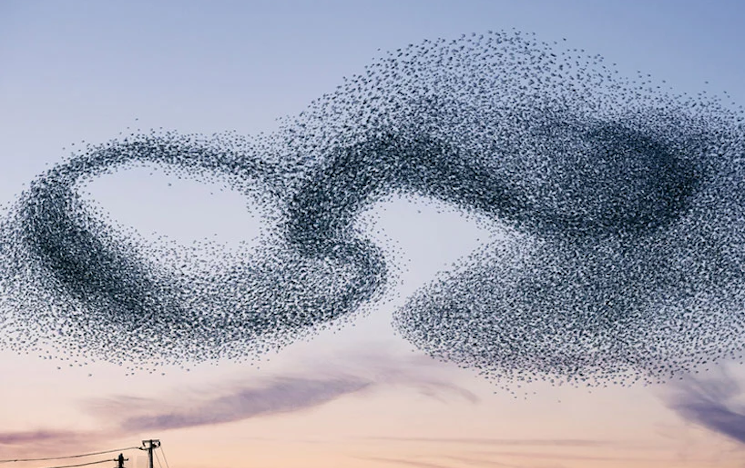
Complex Systems
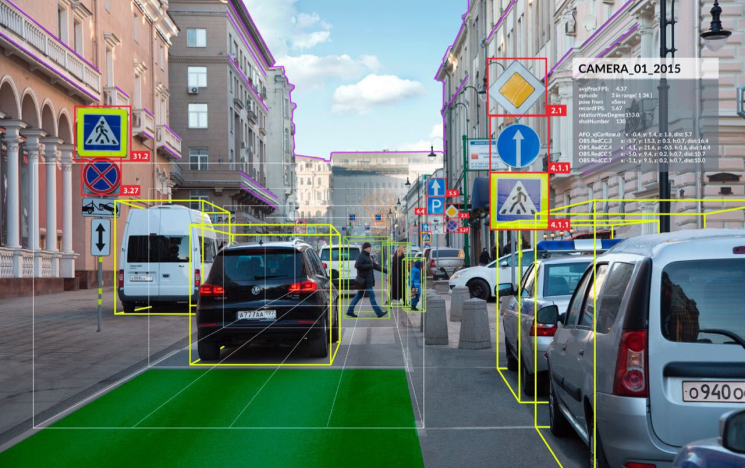
Computer Vision
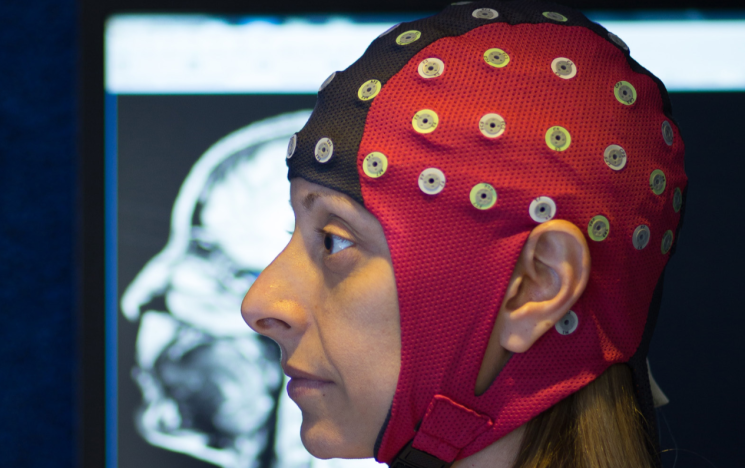
Consciousness
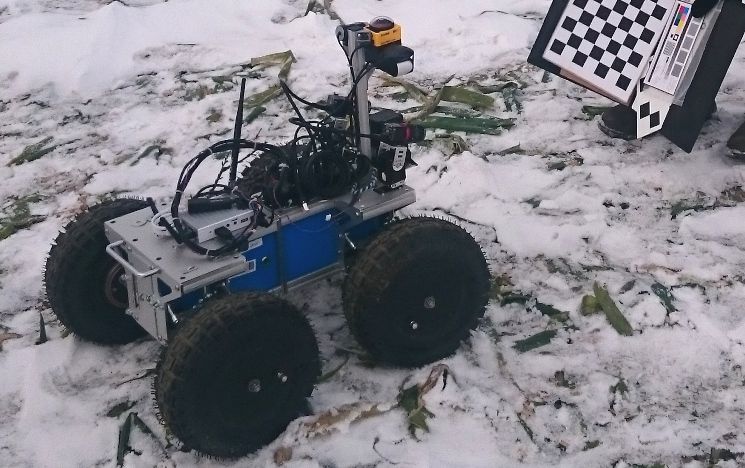
Embodied AI
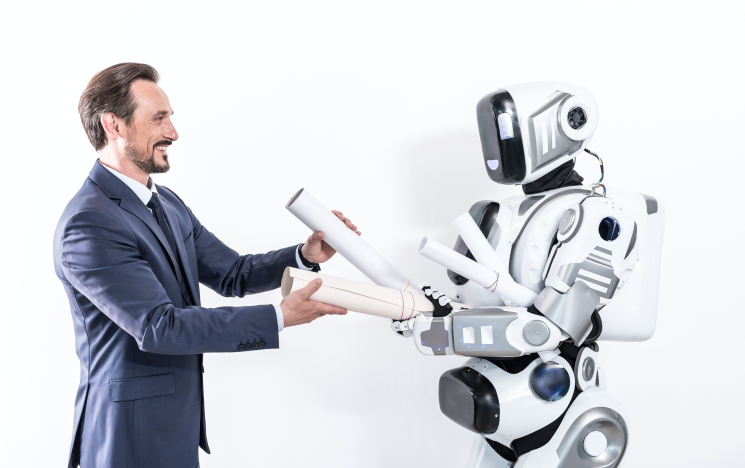
Human and Social AI
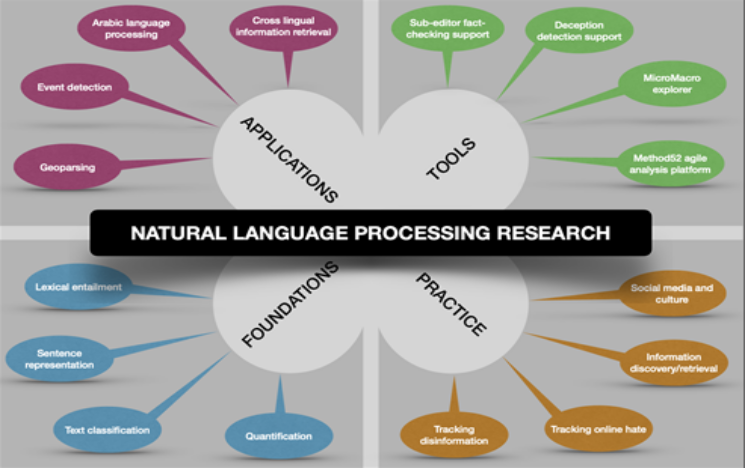