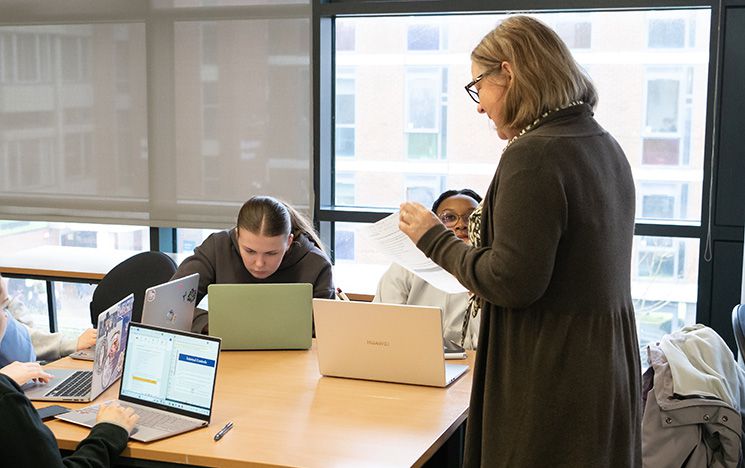
Study
Explore our courses.
Our work draws on management, economics, science, technology and innovation studies, to make an impact on the future of people and institutions the world over. We study the issues that matter, in order to transform our world and your future.
Join us at the University of Sussex Business School.
Change starts here.
[Violin and piano orchestral music fades up and plays throughout the rest of the video]
How can we bring about climate and energy justice?
How can digital transformation at work be inclusive?
How do we create sustainable supply chains?
I want to drive the transition to green energy.
I want to reform policy-making.
I want to inspire the next generation of visionary leaders.
Are you ready to tackle the defining challenges of our time?
Our top ranked University of Sussex Business School is your launch pad.
Our pioneering research drives positive global change.
We empower responsible business leaders
through focus on global social responsibility and sustainability values.
Equip yourself to solve real-world problems with innovative solutions.
Join our inclusive and supportive community.
Together, let's shape the future of policy, practice, and people.
Change starts here.
Explore our courses.
Discover the impact of our academic activities.
Strengthen your own venture or share expertise.
Understand our history and what we stand for at the Business School.
Explore our departments, get a sense of our research, and see the staff who who will be teaching and supporting you.
Read stories from our community about real-world learning experiences, expert insights, and impact making research.
Keep up to date with our Business School events, including webinars, workshops, guest speakers and seminars.
See all rankings and affiliations
The REF 2021 results confirm the University of Sussex Business School as one of the UK’s foremost producers of world-leading and internationally excellent research tackling the great societal challenges of our time.
Our new research magazine 'Boundless' gives an insight into the latest innovative research here at the Business School.
Find out more about REF 2021 at the University of Sussex
See full Business School news listing
At the University of Sussex Business School we are working to tackle some of the key challenges facing modern government, industry and civil society - from low-carbon energy systems and international trade to innovation policy and digital practices at work. Many projects are investigating different ways to meet the UN's Sustainable Development Goals.
[Violin and piano orchestral music fades up and plays throughout the rest of the video]
[On screen text - ‘Prof Paul Nightingale – Associate Dean of Research, University of Sussex’]
The world is increasingly complex, interconnected, fast moving, and that creates both problems and opportunities for society, for governments, and for people’s lives.
Globally we're facing constantly evolving challenges. Research is an incredibly powerful tool for change in society, just small improvements in our understanding can have really fundamental changes in outcomes.
Here in the Business School our research is driven by big global challenges, we're not here to do theory for theories sake, we're trying to create solutions.
[On screen text - ‘Prof Michael Gasiorek – UK Trade Policy Observatory and Co-Director, Centre for Inclusive Trade, University of Sussex’]
Trade affects all of our lives in major ways. The world trading system is facing some major challenges to do with geopolitical conflict, climate change, the rise of the digital economy and supply chain resilience.
The work we do is very much focused on understanding how trade affects people. It's important that policy takes into account those equity issues, those distributional issues and is inclusive.
If you're going to do research that has an impact, you need to collaborate. We need to bring different groups of people together, we need to bring different academic voices from different academic disciplines to find new solutions.
[On screen text - ‘Prof Mari Martiskainen – Director, Energy Demand Research Centre and Co-Director, Sussex Energy Group, University of Sussex’]
Climate change is one of the biggest challenges that we face in today's world. We have a chance still to do something about it.
We have a large number of researchers looking at issues such as industrial decarbonisation, energy demand reduction, and community level action.
We also look at things like climate finance, making sure that we have the right business models that can actually get us to net zero by 2050.
Some of the solutions that we are looking at include policy measures, what are the sort of technological solutions that we need in energy demand reduction, and lastly, what are the behavioural messages that we might need in terms of people changing the way they use energy going forward.
[On screen text - ‘Prof Jacqueline O’Reilly – Co-Director, Digital Futures at Work Research Centre, University of Sussex’]
Digital transformation of work is going to be one of the biggest disruptive changes we have, equivalent to the Industrial Revolution.
What we're trying to understand is how do firms adopt these technologies and why they don't, who are connected or disconnected and how this affects workers in terms of the quality of their jobs.
Leadership, literacy and infrastructure are the really key areas we need to focus on.
The ultimate goal is to inform key actors how to develop a digital transformation that is inclusive and constructive so that it will not lead to increased forms of inequality.
[On screen text - ‘Prof Michael Gasiorek – UK Trade Policy Observatory and Co-Director, Centre for Inclusive Trade, University of Sussex’]
What we really care about is our work having a tangible impact on policy and policy decisions.
We're doing it in order to improve the way society functions and the decisions taken by governments.
[On screen text - ‘Prof Paul Nightingale – Associate Dean of Research, University of Sussex’]
We are committed to using our research on delivering better outcomes for people, for policy and for practice, to create a fairer society and a more equal and prosperous world.